The Untold Truth About Meta's LLAMA 4: Benchmarks, Controversy, and Performance
Explore the untold truth behind Meta's LLAMA 4 AI model. Discover the benchmarking controversies, performance analysis, and industry insights that shed light on the model's capabilities and development.
٩ أبريل ٢٠٢٥
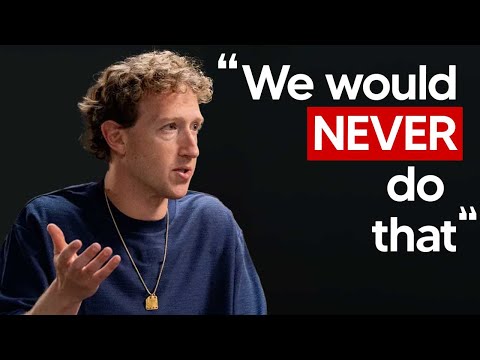
Discover the truth behind the hype surrounding Meta's latest AI model, Llama 4. This blog post delves into the recent revelations and controversies surrounding the model's performance, offering a balanced perspective on the ongoing debate within the AI industry.
The Questionable Benchmarks for Llama 4
The Potential Tampering with Llama 4 Benchmarks
Meta's Response to the Mixed Quality of Llama 4
Conflicting Reports on Llama 4's Performance
Analyzing Other Benchmarks for Llama 4
Conclusion
The Questionable Benchmarks for Llama 4
The Questionable Benchmarks for Llama 4
The release of Llama 4 by Meta has been surrounded by controversy, with concerns raised about the model's benchmarks not living up to the hype. One of the key issues is that Llama 4 was released without a technical paper, which raises questions about the transparency of the model's internal workings and training process.
Some have argued that this lack of transparency is further evidence that Meta may have tampered with the benchmarks to achieve better results. However, others, including the author, believe that the model is actually quite decent, despite the mixed reports on its performance.
The author presents evidence from various sources, including an anonymous post on Reddit that suggests Meta's Gen AI organization was in "panic mode" due to the release of Deepseek V3, which reportedly outperformed Llama 4 in benchmarks. This post also raises concerns about the high compensation of Gen AI leaders, questioning whether the organization is overspending on talent.
Additionally, the author discusses a statement from Ethan Molik, an AI professor, who has observed differences between the Llama 4 model used in benchmarks and the version released to the public. Molik suggests that the benchmark results may have been manipulated to appear more favorable to humans.
The author also examines other benchmarks, such as the MMLU Pro and GPQA Diamond, where Llama 4's performance has been questioned. However, the author notes that the model's performance may vary depending on the specific use case and that their personal experience with Llama 4 has been positive.
Overall, the author acknowledges the ongoing drama and concerns surrounding the Llama 4 benchmarks, but remains cautiously optimistic about the model's capabilities. The author encourages readers to test the model themselves and share their experiences in the comment section.
The Potential Tampering with Llama 4 Benchmarks
The Potential Tampering with Llama 4 Benchmarks
The release of Llama 4 has been a controversial one, with reports of the model's benchmarks not living up to the hype. There are concerns that Meta may have tampered with the benchmarks to present better results.
One of the key issues is that Llama 4 was released without a technical paper, which raises questions about the transparency of the model's internal workings and training techniques. This lack of information has led some to speculate that Meta may have intentionally manipulated the benchmarks.
Further evidence of potential tampering comes from an anonymous post on Reddit, which suggests that Meta's Gen AI organization was in "panic mode" after the release of Deepseek V3, which reportedly outperformed Llama 4 in benchmarks. The post also mentions that Meta's leadership was concerned about justifying the massive costs of the Gen AI program, leading to potential pressure to produce favorable benchmark results.
Additionally, an AI professor, Ethan Molik, has noted that there were differences between the Llama 4 model used in the benchmarks and the version released to the public. This discrepancy raises concerns about the reliability and transparency of the benchmark results.
Meta has responded to these claims, stating that they would never train on test sets and that the variable quality seen in the model is due to the need to stabilize implementations. However, there are still lingering doubts about the integrity of the Llama 4 benchmarks.
Ultimately, the controversy surrounding Llama 4 highlights the need for greater transparency and accountability in the AI industry. As the field continues to advance, it is crucial that benchmark results are reliable and accurately reflect the capabilities of the models being developed.
Meta's Response to the Mixed Quality of Llama 4
Meta's Response to the Mixed Quality of Llama 4
Meta has acknowledged the reports of mixed quality across different services for the Llama 4 model. They stated that they released the model as soon as it was ready, and it will take several days for the public implementations to get stabilized. Meta also refuted the claims that they trained on test sets, stating that this is simply not true and they would never do that.
According to Meta, the variable quality people are seeing is due to the need to stabilize implementations, and they believe the Llama 4 models are a significant advancement. They are looking forward to working with the community to unlock the value of these models.
Meta's response suggests that they are aware of the issues surrounding the Llama 4 release and are committed to addressing them. They emphasize the need for further stabilization and optimization of the public implementations, while also defending the integrity of the model's training process.
Conflicting Reports on Llama 4's Performance
Conflicting Reports on Llama 4's Performance
The release of Llama 4 by Meta has been surrounded by controversy, with conflicting reports on its performance. While some have praised the model's capabilities, others have raised concerns about potential issues with the benchmarks and the model's actual performance.
One of the key points of contention is the lack of a technical paper accompanying the model's release. This has led to speculation that Meta may have tampered with the benchmarks to achieve better results. Additionally, there are reports of differences between the model used in the benchmarks and the one released to the public, raising questions about the transparency and accuracy of the results.
Further evidence of potential issues comes from an anonymous post on Reddit, which suggests that Meta's Gen AI organization was in "panic mode" due to the release of Deepseek V3 and the performance of an unknown Chinese company. The post also raises concerns about the high compensation of Gen AI leaders and the potential need to reevaluate the organization's structure.
Ethan Molik, an AI professor, has also noted differences between the Llama 4 model used in the benchmarks and the one released to the public, stating that the released model appears to be less capable. This raises concerns about the reliability of the benchmark results and the transparency of Meta's process.
Despite these concerns, Meta has responded by acknowledging the reports of mixed quality across different services, stating that they are working to stabilize the implementations and unlock the value of the Llama 4 models. However, the company has also firmly denied any claims of training on test sets, stating that this is simply not true.
Ultimately, the performance of Llama 4 remains a topic of debate, with conflicting reports and evidence suggesting both positive and negative aspects of the model. As the AI industry continues to evolve, it is crucial that companies maintain transparency and integrity in their processes to ensure the reliable and trustworthy development of these powerful technologies.
Analyzing Other Benchmarks for Llama 4
Analyzing Other Benchmarks for Llama 4
While the benchmarks for Llama 4 have been a topic of much discussion, it's important to look at the model's performance across a variety of evaluations to get a more comprehensive understanding.
One benchmark that provides expert-driven rankings of large language models is the CLLM (Curated Language Model) Leaderboard, developed by Scale AI's Safety Evaluations and Alignment Lab. These evaluations use private datasets to ensure unbiased results, and cover domains such as coding, instruction following, math, and multilingualism.
When examining the CLLM leaderboard, it's worth noting that the Llama 4 Maverick model has a potential contamination warning, indicating that the model was evaluated after the public release of the "Humanity's Last Exam" dataset, potentially allowing the model builders access to the prompts and solutions. This caveat should be considered when interpreting the model's performance on this particular benchmark.
Similarly, other CLLM evaluations, such as the Enigma and Multi-Challenge assessments, also carry potential contamination warnings for the Llama 4 Maverick model. This suggests that the model's performance on these private datasets may not be entirely representative of its true capabilities.
Despite these caveats, the CLLM leaderboard can still provide valuable insights into Llama 4's performance relative to other large language models. By considering the model's scores across multiple expert-curated evaluations, a more nuanced understanding of its strengths and weaknesses can be gained.
It's important to note that benchmarking large language models is a complex and evolving field, and the results can be influenced by various factors, including the specific datasets, evaluation methodologies, and the model's training and fine-tuning processes. As such, it's crucial to approach these benchmarks with a critical eye and to consider multiple sources of information when assessing the capabilities of Llama 4 and other AI models.
Conclusion
Conclusion
The recent release of Llama 4 by Meta has been a topic of much discussion and controversy within the AI community. While the benchmarks for the model may not have lived up to the initial hype, it's important to consider the various factors at play.
One key issue is the lack of a technical paper accompanying the model's release, which has raised concerns about transparency and the ability to fully understand the model's internal workings. Additionally, there have been reports of discrepancies between the benchmarks used for testing and the actual performance of the released model, leading some to question the integrity of the testing process.
However, it's also important to note that the performance of an AI model can be highly dependent on the specific use case and implementation. Anecdotal evidence suggests that Llama 4 may perform well in certain applications, such as social media automation, while struggling in others, like coding or app development.
Ultimately, the true capabilities of Llama 4 remain a subject of debate and ongoing investigation. As the AI industry continues to evolve rapidly, it's crucial that companies like Meta maintain transparency and integrity in their model development and testing processes. Only then can the community have confidence in the accuracy and reliability of the benchmarks and results.
التعليمات
التعليمات