Major Llama Drama: Meta's Controversial Llama 4 Release Analyzed
Dive into the controversy surrounding Meta's Llama 4 release and its customized version optimized for the LM Arena leaderboard. Explore the model's performance on various benchmarks and industry experts' perspectives on the release.
10. April 2025
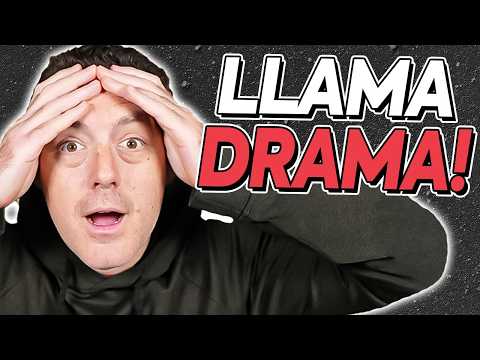
Discover the captivating story behind the latest AI model release, Llama 4, and explore the intriguing drama surrounding its performance on the LM Arena leaderboard. This blog post delves into the nuances of model optimization, benchmarking, and the importance of transparency in the AI community.
The Truth About Llama 4's Controversial Scoring on the LM Arena Leaderboard
Why Llama 4's Performance on Other Benchmarks Raises Questions
The Complexity of Launching a Major AI Model Like Llama 4
Conclusion
The Truth About Llama 4's Controversial Scoring on the LM Arena Leaderboard
The Truth About Llama 4's Controversial Scoring on the LM Arena Leaderboard
Meta, the company behind the Llama language models, has recently faced criticism for the performance of their Llama 4 model on the LM Arena leaderboard. The issue stems from the fact that Meta created a custom version of the Llama 4 model specifically optimized for the LM Arena benchmark, which led to the model scoring highly on that particular leaderboard.
The LM Arena leaderboard is a human evaluation benchmark, where users are presented with two different model outputs and asked to choose the one they prefer. This type of evaluation is different from traditional benchmarks, which typically involve a set of predefined questions or tasks that the model is tested against.
Meta's decision to create a custom version of Llama 4 for the LM Arena leaderboard has been met with mixed reactions. On one hand, they were transparent about this approach and disclosed the use of the optimized model in their report. However, some argue that this could be considered a form of "cheating," as the model is not being evaluated on its general capabilities but rather on its ability to appeal to human preferences.
The performance of the standard Llama 4 model on other benchmarks, such as the Ader Polyglot coding benchmark, has been less impressive, highlighting the potential limitations of the model's capabilities outside of the LM Arena scenario.
Ultimately, the debate surrounding Llama 4's performance on the LM Arena leaderboard raises important questions about the role of human evaluation in AI benchmarking and the potential for model providers to optimize their models for specific tasks or metrics. As the field of AI continues to evolve, it will be crucial for the community to maintain a focus on transparency, fairness, and the development of robust and generalizable models.
Why Llama 4's Performance on Other Benchmarks Raises Questions
Why Llama 4's Performance on Other Benchmarks Raises Questions
While Llama 4's performance on the LM Arena leaderboard was impressive, its results on other benchmarks raise some concerns. The Llama 4 Maverick model, which was specifically optimized for conversationality, did not score as highly on other evaluations.
On the Ader Polyglot coding benchmark, the Llama 4 Maverick model scored only around 16%, significantly lower than the top-performing models like Gemini 2.5 Pro, which scored over 70%. This suggests that the Llama 4 Maverick model may have been overfitted for the LM Arena leaderboard, sacrificing performance on other tasks.
Similarly, in the independent evaluations conducted by Artificial Analysis, the Llama 4 models performed "quite well" but were compared to non-reasoning models, which Nathan Lambert argues is not an appropriate comparison. When reasoning and non-reasoning tasks are evaluated separately, the Llama 4 models may not perform as strongly.
Furthermore, the Llama 4 models struggled in the long-context benchmark on fiction.live, where they scored significantly lower than the top-performing Gemini 2.5 Pro model. This raises questions about the Llama 4 models' ability to handle long-form, contextual tasks.
Overall, the mixed performance of the Llama 4 models on various benchmarks suggests that the model may have been optimized specifically for the LM Arena leaderboard, potentially at the expense of more general capabilities. This raises concerns about the model's true capabilities and the transparency of Meta's development process.
The Complexity of Launching a Major AI Model Like Llama 4
The Complexity of Launching a Major AI Model Like Llama 4
The release of Llama 4 by Meta has been a complex and controversial event in the world of artificial intelligence. While the model itself represents a significant advancement, the launch has been marked by a number of challenges and criticisms.
One of the key issues is the use of a custom version of the model specifically optimized for the LM Arena leaderboard. This custom version, which Meta disclosed, scored highly on the leaderboard but did not perform as well on other benchmarks. This has led to accusations of "cheating" and concerns about the model's true capabilities.
Additionally, the release timing and lack of comprehensive benchmarking have raised eyebrows. The model was launched on a Saturday, which is an unusual choice for a major product release, and the initial benchmarks were limited to the "needle in a haystack" test, leaving many other important metrics unaddressed.
Independent evaluations of the model have also yielded mixed results, with some reports ranging from "medium bad" to "confusing." This has led to questions about the model's overall quality and performance.
Furthermore, the cultural challenges within Meta's Genai organization, including the departure of the head of AI research just days before the launch, have added to the complexity of the situation.
Despite these challenges, there is still optimism about the potential of the Llama 4 models. Meta's own representatives have acknowledged the need for further stabilization and tuning of the implementations, and have expressed a commitment to working with the community to unlock the value of these models.
In the end, the launch of Llama 4 highlights the inherent complexity of introducing a major AI model to the world. It requires careful planning, comprehensive benchmarking, and a commitment to transparency and collaboration with the broader AI community. As the dust settles, it will be interesting to see how Llama 4 and its successors evolve and contribute to the ongoing advancements in artificial intelligence.
Conclusion
Conclusion
The release of Llama 4 by Meta has been a topic of much discussion and debate within the AI community. While the model has shown impressive performance on certain benchmarks, particularly the LM Arena leaderboard, it has also faced criticism for the use of a custom version optimized for conversationality.
The key points to consider are:
-
LM Arena is not a true benchmark: Unlike traditional benchmarks with a fixed set of questions, LM Arena relies on human evaluators to choose between two model outputs. This makes it more susceptible to models being optimized for human preference rather than general performance.
-
Meta's disclosure of the custom model: While Meta did disclose the use of a custom version of Llama 4 for the LM Arena leaderboard, this raises questions about the transparency and fairness of the process.
-
Performance on other benchmarks: When evaluated on more traditional coding and reasoning benchmarks, such as the Ader Polyglot benchmark, Llama 4 has not performed as strongly, highlighting the potential for overfitting on the LM Arena task.
-
Ongoing development and improvement: As noted by Meta's AI lead, the Llama 4 models are still in the early stages of development, and further iterations and optimizations are likely to improve their overall performance across a range of tasks.
In conclusion, the Llama 4 release has been a complex and nuanced event, with both positive and negative aspects. While the model's strong performance on the LM Arena leaderboard is noteworthy, the use of a custom version and the mixed results on other benchmarks raise valid concerns about transparency and fairness. As the Llama 4 models continue to evolve, it will be important for the AI community to closely monitor their development and performance across a diverse set of tasks and benchmarks.
FAQ
FAQ