Qwen-3: The Innovative Hybrid AI Model with Enhanced Reasoning and Coding Capabilities
Explore the groundbreaking Qwen-3 hybrid AI model, boasting enhanced reasoning and coding capabilities. Discover its innovative features, including on-demand thinking mode and expanded language support. Unlock the potential of this cutting-edge AI technology.
2025年4月29日
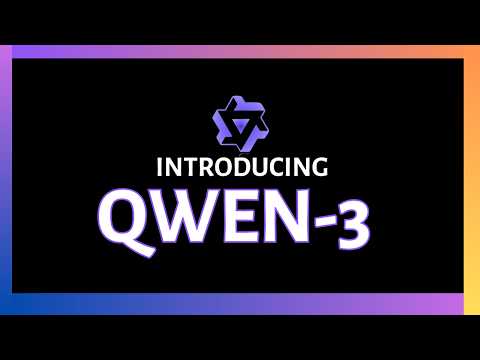
Discover the power of Qwen-3, the latest AI model that delivers exceptional performance in a compact package. Explore its innovative hybrid architecture, advanced coding and agential capabilities, and seamless integration with MCPS. This model is poised to revolutionize your workflows, offering unparalleled versatility and efficiency.
Qwen-3: The Hybrid Architecture with Thinking or Reasoning on Demand
Benchmark Performance Comparison: Qwen-3 vs OpenAI GPT-4 and DeepSeek R1
The Power of Smaller Models: Qwen-3's Impressive Capabilities
Hybrid Thinking Mode: Enabling or Disabling Reasoning on Demand
Reasoning Capabilities: Enhancing Complex Problem-Solving
Multimodal Support and Agentic Capabilities of Qwen-3
Qwen-3's Innovative Pre-Training and Post-Training Process
Using Qwen-3: Enabling or Disabling Thinking in Your Workflows
Conclusion
Qwen-3: The Hybrid Architecture with Thinking or Reasoning on Demand
Qwen-3: The Hybrid Architecture with Thinking or Reasoning on Demand
The Qwen 3 models are the latest release from Qwen, featuring a hybrid architecture that allows for on-demand thinking or reasoning capabilities. These models demonstrate impressive performance, particularly considering their relatively smaller size compared to other large language models.
The key highlights of the Qwen 3 models include:
-
Hybrid Thinking Mode: The models can be configured to either enable or disable the thinking/reasoning process, allowing for quick responses for simpler tasks or more in-depth, step-by-step thinking for complex problems.
-
Improved Benchmarks: The larger 235 billion parameter Mixture of Experts (MOE) model outperforms the OpenAI GPT-3.5 and is comparable to the Gemini 2.5 Pro on various benchmarks. The 32 billion parameter dense model also outperforms the DeepSeek R1 on several key metrics.
-
Increased Context Window: The dense models have a context window of up to 128,000 tokens, while the MOE models can handle up to 128,000 tokens, making them suitable for long-form tasks.
-
Multimodal and Multilingual Capabilities: The models support 119 different languages, including Afro-African and Eastern languages, and have multimodal capabilities.
-
Improved Coding and Agentic Capabilities: The models demonstrate strong coding abilities and support for MCPS (Model-Calling Primitive Sequences), allowing for tool-assisted reasoning and problem-solving.
The performance improvements in the Qwen 3 models are attributed to the use of high-quality synthetic data, generated using the previous generation of Qwen models, as well as a four-stage post-training process that enables the hybrid thinking/non-thinking capabilities.
Overall, the Qwen 3 models represent a significant advancement in large language model architecture and capabilities, setting a new benchmark for the industry.
Benchmark Performance Comparison: Qwen-3 vs OpenAI GPT-4 and DeepSeek R1
Benchmark Performance Comparison: Qwen-3 vs OpenAI GPT-4 and DeepSeek R1
Based on the benchmarks presented, the Qwen-3 models demonstrate impressive performance compared to OpenAI GPT-4 and DeepSeek R1:
- The largest Qwen-3 model, a 235 billion parameter Mixture of Experts (MOE) model, outperforms the OpenAI GPT-4 model on several key benchmarks.
- The 32 billion parameter dense Qwen-3 model also matches or beats the performance of the OpenAI GPT-4 model, while being significantly smaller in size.
- The smaller 30 billion parameter MOE Qwen-3 model outperforms the previous generation 32 billion Qwen model, which was nearly 10 times larger.
- The 22 billion parameter dense Qwen-3 model is reported to be comparable to the Gemini 2.5 Pro model on various benchmarks, despite its much smaller size.
- On coding benchmarks, the locally-runnable Qwen-3 models are shown to outperform the GPT-4 model.
These results suggest that Qwen-3 has been able to pack a significant amount of performance into relatively smaller model sizes compared to previous generation models and competitors. The hybrid architecture that enables on-demand thinking or reasoning capabilities appears to be a key factor in this improved efficiency.
The Power of Smaller Models: Qwen-3's Impressive Capabilities
The Power of Smaller Models: Qwen-3's Impressive Capabilities
The new Qwen-3 models have set a new benchmark for performance in a smaller package. These hybrid models offer the ability to enable or disable "thinking" or reasoning on demand, thanks to their innovative four-stage learning process.
The key features that set these models apart are the hybrid thinking mode and the impressive coding and agentic capabilities. The models can switch between a "thinking mode" that takes time to respond with step-by-step reasoning, and a "non-thinking mode" that provides quick, near-instant responses. This flexibility is enabled by the four-stage post-processing approach.
The benchmarks show that the larger 235-billion-parameter mixture-of-experts model outperforms the OpenAI GPT-3.1 and is comparable to the Gemini 2.5 Pro on several key metrics. Even the smaller 32-billion-parameter dense model is competitive with GPT-3.1 and beats the DeepSeek R1 on various benchmarks.
The models' performance is attributed to the high-quality synthetic data used in pre-training, which was generated using the previous generation of Qwen models. This approach of leveraging earlier models to improve the next generation is a trend seen in the industry as data sources become scarce.
The post-training process is where the models truly shine. By going through a four-step fine-tuning process, the models learn to enable and disable the thinking mode, as well as enhance their exploration and exploitation capabilities for complex reasoning tasks.
These Qwen-3 models are available under the Apache 2.0 license and offer support for 119 languages, including Afro-African and Eastern languages. The models' coding and agentic capabilities, along with the native support for MCPS, make them highly versatile for a wide range of applications.
Hybrid Thinking Mode: Enabling or Disabling Reasoning on Demand
Hybrid Thinking Mode: Enabling or Disabling Reasoning on Demand
The key feature that sets the Quen 3 models apart is their hybrid thinking mode. This is the first time openweight models have the capability to enable or disable thinking on demand.
There are two modes available:
-
Thinking Mode: In this mode, the model takes time to respond, providing a step-by-step chain of thought before delivering the final answer. This is great for complex reasoning tasks.
-
Non-Thinking Mode: In this mode, the model provides quick, near-instant responses, suitable for simpler questions where speed is more important than depth.
The ability to toggle between these two modes is controlled by a single hyperparameter. This allows users to leverage the model's reasoning capabilities when needed, while also benefiting from its speed for more straightforward queries.
Enabling the thinking mode has been shown to improve performance on complex problems, such as the AIM 24 and 25 benchmarks. However, the impact may vary depending on the nature of the task, so it's recommended to run your own tests to determine the optimal settings for your use case.
The hybrid thinking capability is made possible by the model's four-stage post-training process, which integrates the non-thinking mode into the original reasoning-focused model. This innovative approach allows users to access both modes within a single model, providing flexibility and efficiency.
Reasoning Capabilities: Enhancing Complex Problem-Solving
Reasoning Capabilities: Enhancing Complex Problem-Solving
The new Quen 3 models introduce a groundbreaking hybrid architecture that enables on-demand thinking or reasoning capabilities. This innovative feature sets these models apart, allowing users to toggle between two distinct modes: thinking mode and non-thinking mode.
In the thinking mode, the model takes time to respond, providing a step-by-step chain of thought before delivering the final answer. This is particularly beneficial for complex reasoning tasks, where the model's ability to engage in deeper analysis can lead to more accurate and insightful solutions.
On the other hand, the non-thinking mode offers quick, near-instant responses, making it suitable for simpler questions where speed is more important than depth. The ability to seamlessly switch between these modes within a single model is a significant advancement, providing users with the flexibility to tailor the model's behavior to their specific needs.
The reported results demonstrate the effectiveness of the thinking mode, with significant performance improvements on benchmarks like AIM 24 and 25. By enabling the model to utilize more tokens for its thought process, the accuracy can increase by up to 100%, showcasing the value of this reasoning capability.
However, it's important to note that the impact of longer thinking may vary depending on the nature of the task. As highlighted, there have been reports from the ARC or ARC AGI V2 benchmark where extended thinking did not result in more accurate answers, suggesting that the model may have been able to find the correct solutions through shorter thought processes. Therefore, it is recommended to conduct your own tests and experiments to determine the optimal balance between thinking time and performance for your specific use cases.
Overall, the integration of this hybrid thinking mode is a significant advancement in the field of large language models, empowering users to leverage the model's reasoning capabilities for complex problem-solving tasks while maintaining the efficiency of quick responses for simpler queries.
Multimodal Support and Agentic Capabilities of Qwen-3
Multimodal Support and Agentic Capabilities of Qwen-3
The Qwen-3 models released by Qwen AI are highly capable and versatile, offering several key features that set them apart:
-
Multimodal Support: The Qwen-3 models support 119 different languages, including Afro-African languages and Eastern languages like Turkish and Arabic. This broad language support makes them suitable for a wide range of applications and users.
-
Coding and Agentic Capabilities: The Qwen-3 models have strong coding capabilities and agentic capabilities, allowing them to perform complex tasks. They natively support MCPS (Model-Centric Programming Systems), which enables the models to use various tools and functions during their thought process to solve problems.
-
Hybrid Thinking Mode: One of the key features of the Qwen-3 models is the ability to enable or disable the "thinking" mode on demand. In the thinking mode, the model takes time to respond with a step-by-step chain of thought, which is beneficial for complex reasoning tasks. In the non-thinking mode, the model provides quick, near-instant responses, suitable for simpler questions where speed is more important than depth. This hybrid architecture allows users to choose the appropriate mode based on their specific needs.
-
Improved Performance: The Qwen-3 models demonstrate impressive performance, often outperforming larger models like GPT-4 and DeepSeek R1 on various benchmarks, including coding tasks. This is achieved through the use of high-quality synthetic data and the leveraging of the previous generation of Qwen models to generate and improve the training data.
-
Flexible Deployment: The Qwen-3 models are released under the Apache 2.0 license, making them accessible to a wide range of users. The models are also optimized for deployment in high-throughput environments, with the recommendation to use tools like VLM or SG Lang for production use cases.
Overall, the Qwen-3 models showcase the continued advancements in large language models, offering a unique combination of multimodal support, agentic capabilities, and hybrid thinking modes that can be tailored to various application needs.
Qwen-3's Innovative Pre-Training and Post-Training Process
Qwen-3's Innovative Pre-Training and Post-Training Process
The key to Qwen-3's impressive performance lies in its innovative pre-training and post-training process.
The pre-training process consisted of three stages:
- Initial pre-training on over 30 trillion tokens with a context window of 4,000 tokens.
- Improving the dataset by increasing the proportion of knowledge-intensive tasks such as STEM, coding, and reasoning. The model was then pre-trained for an additional 5 trillion tokens.
- Using high-quality long-context data to extend the context window to 32,000 tokens.
The post-training process is where Qwen-3 truly shines. It involves a four-step process:
- Fine-tuning the model using long chain-of-thought data, focusing on tasks like mathematics, coding, logical reasoning, and STEM problems. This teaches the model how to think about these complex problems.
- Scaling up computational resources for reinforcement learning (RL) and using rule-based rewards to enhance the model's exploration and exploitation capabilities. This further strengthens the model's reasoning abilities.
- Integrating non-thinking capabilities into the thinking model by fine-tuning on a combination of long-context chain-of-thought data and commonly used instruction-tuning datasets. This allows the model to disable thinking when appropriate.
- Applying general RL across more than 20 domains to strengthen the model's capabilities and correct any undesired behavior. This serves as an alignment process.
The result of this innovative pre-training and post-training process is a highly capable hybrid model that can toggle between thinking and non-thinking modes, delivering impressive performance on a wide range of tasks, including coding, reasoning, and STEM-related problems.
Using Qwen-3: Enabling or Disabling Thinking in Your Workflows
Using Qwen-3: Enabling or Disabling Thinking in Your Workflows
The key feature that sets the Qwen-3 models apart is their hybrid thinking mode. These models allow you to enable or disable the thinking process on demand, providing flexibility to cater to different use cases.
In the "thinking mode," the model takes time to respond, providing a step-by-step chain of thought before delivering the final answer. This is particularly useful for complex reasoning tasks where depth of analysis is crucial. On the other hand, the "non-thinking mode" offers quick, near-instant responses, making it suitable for simpler questions where speed is more important than depth.
To enable or disable the thinking process, you can use a single hyperparameter. This allows you to seamlessly switch between the two modes within the same model, without the need to work with separate models.
The benefits of the thinking mode have been demonstrated across various benchmarks. For example, on the AIM 24 and 25 benchmarks, enabling the thinking process can lead to almost a 100% improvement in performance compared to the non-thinking mode. Similarly, the thinking tokens have shown to be beneficial for coding tasks as well.
To use the thinking or non-thinking mode in your workflows, you can leverage the Transformer package. By setting the enable_thinking
flag to True
or False
, you can easily switch between the two modes. This flexibility allows you to tailor the model's behavior to your specific requirements, ensuring optimal performance for your use cases.
Conclusion
Conclusion
The release of Quen 3 models is a significant development in the field of large language models. These models demonstrate impressive performance, with the largest 235 billion parameter model outperforming OpenAI's GPT-3.1 and being comparable to Gemini 2.5 Pro on key benchmarks.
The key feature that sets these models apart is the hybrid thinking mode, which allows users to enable or disable the model's reasoning capabilities on demand. This flexibility is enabled by the four-stage post-training process, which teaches the model to switch between thinking and non-thinking modes.
The models also boast impressive coding and agentic capabilities, with native support for MCPS (Model-Calling Primitive Services). This allows the models to use external tools and services during their reasoning process, further enhancing their problem-solving abilities.
The performance of these smaller models is attributed to the use of high-quality synthetic data, generated using the previous generation of Quen models. This approach of leveraging earlier models to improve the next generation is a promising trend in the field of large language models.
Overall, the Quen 3 models represent a significant advancement in the state of the art, and their hybrid thinking capabilities, coding prowess, and agentic features make them a compelling choice for a wide range of applications.
FAQ
FAQ