Renowned AI Pioneer Warns AGI is Decades Away, Not Years
Renowned AI pioneer Yan Lecun warns that achieving AGI is decades away, not years. He critiques current approaches like LLMs and recommends abandoning generative models in favor of more robust architectures to truly replicate human-level intelligence.
31 de março de 2025
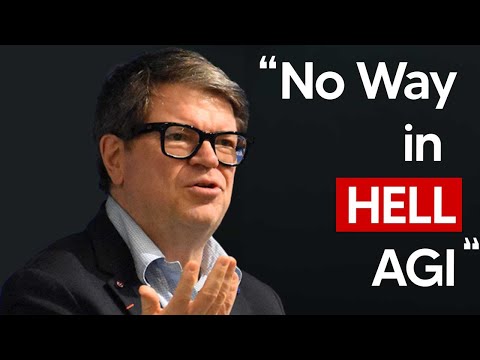
Discover the surprising insights from a renowned AI pioneer who challenges the hype around achieving artificial general intelligence (AGI) in the near future. This blog post delves into the expert's perspective on the limitations of current AI approaches and the critical steps needed to advance towards true human-level intelligence.
The Flaws of Language Models and the Need for a New Approach
The Complexity of Human-Level Intelligence
Yan Lun's Recommendations: Abandoning Generative Models, Contrastive Methods, and Reinforcement Learning
Conclusion
The Flaws of Language Models and the Need for a New Approach
The Flaws of Language Models and the Need for a New Approach
Yan Lun, a renowned AI scientist and one of the "godfathers of AI," has made a bold claim: there is "absolutely no way in hell" that we will achieve AGI (Artificial General Intelligence) within the next two years. He argues that the current approach of relying on large language models (LLMs) is fundamentally flawed and will not lead to human-level AI.
Lun's reasoning is based on a simple calculation. He points out that the amount of information a young child's brain processes in the first four years of life is equivalent to the data used to train the largest LLMs. This suggests that merely training on text data is not enough to achieve AGI, as it fails to capture the rich, embodied understanding of the world that humans possess.
Lun advocates for a radical shift in the approach to AI development. He recommends abandoning generative models, probabilistic models, and reinforcement learning in favor of joint embedding architectures, energy-based models, and model predictive control. He argues that these alternative methods are better suited to capturing the true complexity of human-level intelligence.
Furthermore, Lun cautions against the hype surrounding AGI, warning that the timelines proposed by some industry leaders are unrealistic. He suggests that a more realistic timeline for achieving systems that can "feel like they have human-level intelligence" is five to six years, but even this is contingent on overcoming significant obstacles and challenges.
Lun's perspective is not only informed by his extensive experience in the field but also by a deep understanding of the limitations of current AI approaches. His call for a new direction in AI research and development is a thought-provoking challenge to the prevailing narratives in the industry.
The Complexity of Human-Level Intelligence
The Complexity of Human-Level Intelligence
Yan Lun, a renowned AI scientist and one of the "godfathers of AI," has expressed skepticism about the ability to achieve artificial general intelligence (AGI) within the next two years. He argues that the current approaches, particularly the focus on large language models (LLMs), are unlikely to lead to human-level intelligence.
Lun's key points are:
-
Abandoning Generative Models: He recommends abandoning the popular generative models in favor of joint embedding architectures, which he believes are more suitable for achieving human-level AI.
-
Embracing Energy-Based Models: Lun suggests using energy-based models instead of the intractable probabilistic models currently in use.
-
Shifting from Contrastive to Regularized Methods: He advocates for the use of regularized methods over contrastive methods.
-
Replacing Reinforcement Learning: Lun believes that reinforcement learning should be used as a last resort, and instead, model predictive control should be the preferred approach.
-
Avoiding LLMs for Human-Level AI: Lun strongly advises against working on LLMs if the goal is to achieve human-level AI, as he believes they are not the right path forward.
Lun's perspective highlights the complexity of replicating the human brain's capabilities, which go beyond just predicting the next token in a sequence. He emphasizes the need for AI systems to have a deeper understanding of the real world, including aspects like proprioception, causality, and common sense reasoning.
The Meta CTO's comments further reinforce Lun's views, acknowledging the challenges in building world models that can truly capture the nuances of human intelligence. The CTO suggests that the embodied aspect of intelligence, which involves sensors and robotics, may be a crucial unlock for achieving this level of understanding.
Overall, Lun's recommendations and the insights from the Meta CTO suggest that the path to human-level AI is far more complex than the current approaches may suggest. It will require a fundamental shift in the way AI systems are designed and trained, focusing on more sophisticated architectures and a deeper understanding of the physical world.
Yan Lun's Recommendations: Abandoning Generative Models, Contrastive Methods, and Reinforcement Learning
Yan Lun's Recommendations: Abandoning Generative Models, Contrastive Methods, and Reinforcement Learning
Yan Lun, a renowned AI scientist, has provided several recommendations for those interested in achieving human-level AI. He emphasizes the need to move away from the current popular methods and explore alternative approaches.
-
Abandon Generative Models: Yan Lun suggests abandoning the use of generative models, which are the most popular method today. Instead, he recommends focusing on joint embedding architectures, which predict in representation space rather than using probabilistic models.
-
Abandon Contrastive Methods: Yan Lun advises abandoning contrastive methods in favor of regularized methods. He argues that contrastive methods have been a subject of contention for around 20 years and that regularized methods may be a more effective approach.
-
Abandon Reinforcement Learning: Yan Lun strongly recommends abandoning reinforcement learning, stating that it should only be used as a last resort when the model is inaccurate or the cost function is incorrect. He suggests exploring alternative methods that are more efficient.
-
Avoid Large Language Models (LLMs): If the goal is to achieve human-level AI, Yan Lun advises against working on LLMs. He argues that there is no point in competing with the resources and capabilities of large tech companies in this domain. Instead, he recommends exploring other problems and solutions.
Yan Lun's recommendations challenge the current mainstream approaches in the AI field. He believes that to make progress towards human-level AI, researchers need to explore different architectures and methods that focus on developing real-world intelligence, rather than just predicting the next token. His insights and suggestions provide a thought-provoking perspective on the future direction of AI research.
Conclusion
Conclusion
Yan Lun, a renowned AI scientist and one of the "godfathers of AI," has made a bold claim that achieving AGI (Artificial General Intelligence) within the next two years is simply not possible. He firmly believes that the current approaches, particularly the focus on large language models (LLMs), are not the path forward for human-level AI.
Lun's reasoning is based on a simple calculation that compares the amount of information a young child receives through their visual system in the first four years of life to the data used to train the largest LLMs. He argues that the sheer volume of real-world, multimodal data required to replicate human-level intelligence is far beyond what can be achieved through text-based training alone.
Furthermore, Lun suggests that researchers should abandon the current popular methods, such as generative models, contrastive methods, and reinforcement learning, in favor of alternative approaches like joint embedding architectures, energy-based models, and model predictive control. He believes these alternative methods may be more promising in the pursuit of human-level AI.
Lun's views are not to be taken lightly, given his extensive experience and accomplishments in the field of AI. His warnings about the limitations of LLMs and the need for a fundamental shift in approach are worth considering, even as other prominent figures in the industry maintain more optimistic timelines for AGI.
As the AI community continues to grapple with the challenges of achieving human-level intelligence, Lun's insights provide a thought-provoking perspective that challenges the prevailing narratives and encourages researchers to explore alternative paths forward.
Perguntas frequentes
Perguntas frequentes